Boosting statistical power and context with Multiscale Maps of the Cell
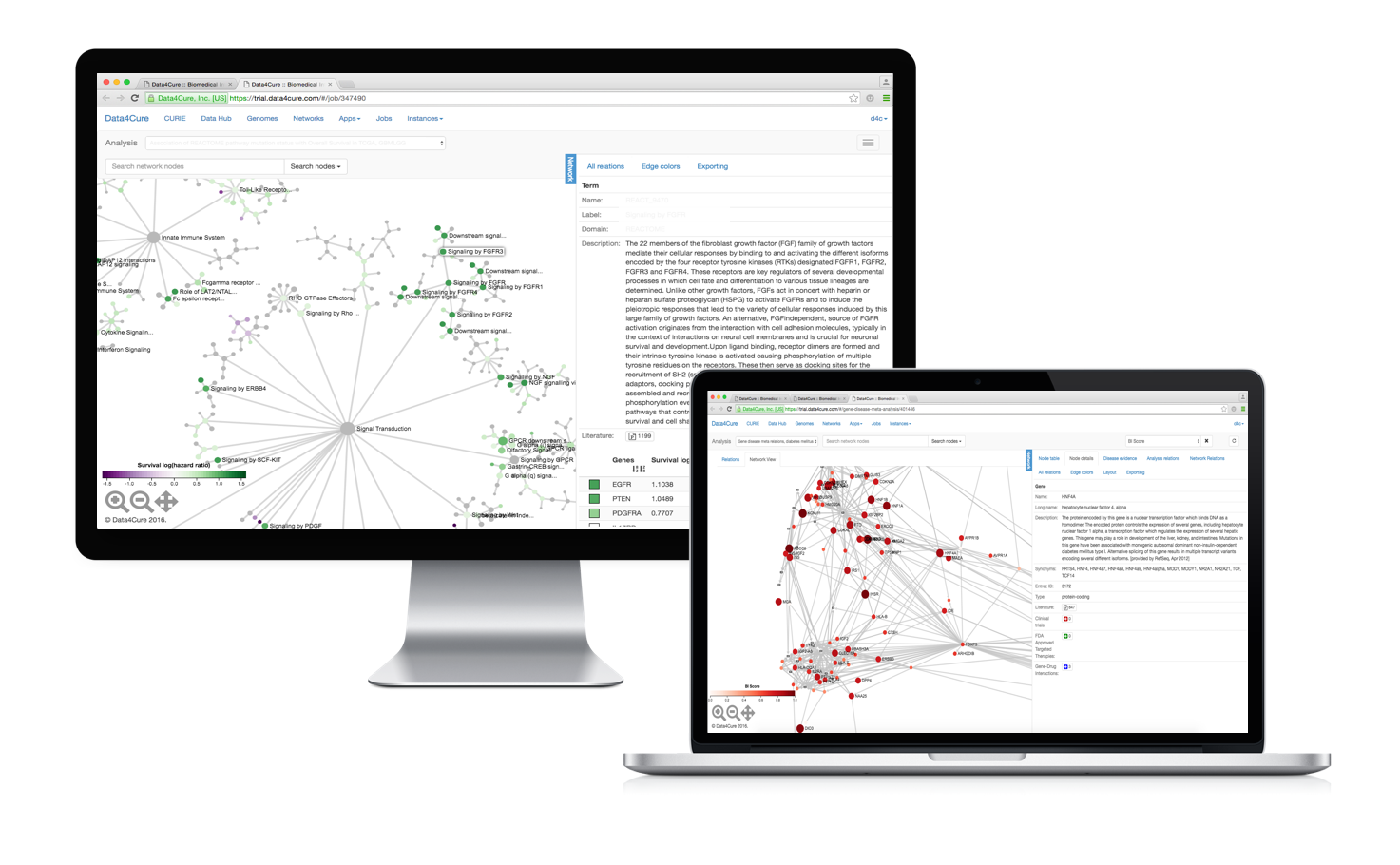
The hierarchy
It is not about components, it’s about the machine. Like many other complex systems, biological machines are hierarchical: proteins interacting in complexes which work as part of pathways forming more general processes and so on.
To better understand disease mechanisms we need to be able to interrogate all levels of the functional hierarchy. It is important to understand when perturbations to different components of the same pathway or process lead to similar phenotypes and when their effects are distinct (component-specific). This has major implications for many practical applications, for example:
1. Deciphering complex genotype-phenotype relationships, e.g. mapping rare variants to common pathways and processes underlying a disease of interest,
2. Predicting the effects of chemical or genetic perturbations, such as gross transcriptional changes in multiple system components.
3. Identifying and optimizing biomarkers towards increased power and specificity, e.g. identifying homogeneous pathway activities and aberrations distinguishing responders from non-responders in clinical trials.
Each of the above tasks can get complex very quickly. One needs to process and integrate large pathway repositories; engineer effective strategies for determining pathway and network activities based on gene-level data; and develop effective tools for visualizing and interrogating the results (pathway analysis results are often complex in their own right).
Our goal for the Multiscale Maps was to simplify the process and make comprehensive systems-level analyses much easier and effective so that they can be one of the go-to tools for many bioinformatics problems.
Multiscale visualization
Data4Cure Maps combine vast repositories of manually-curated pathways but coupled with an unbiased data-driven ontology, the NeXO 2.0 (if you’re interested, take a look at the early papers on NeXO [1, 2, 3]).
To visualize the pathway hierarchies we developed a really nice new multi-scale graph visualization framework. What it does is it allows you to see all the hierarchical system components at once and easily zoom into selected regions of the system to dig deeper.
We tried to make the data, statistics and information associated with each pathway as easily accessible as possible. In fact, molecular data for thousands of biological and medical conditions is available in the system and pre-integrated with the maps (disease areas include oncology, immune system, cardiovascular, metabolic and neurologic conditions). You can start exploring immediately. You can also easily extend the Maps by bringing in your own data for analysis.
Integrating multidimensional data
Maps work with all major types of molecular data not just gene expression. Genetic variants and somatic mutations, gene, miRNA, and protein expression and DNA methylation profiles can be correlated with each other or with available clinical measurements.
For example, the Maps allow you to quickly find disregulated pathways associated with genetic variants and mutations or genetic/chemical perturbations. You can also investigate pathways and processes enriched for mutations (small indel and CNV) and/or epigenetic alterations across various cancer types and find which alterations are significantly associated with clinical variables such as response to treatment and overall survival. The association engine analyzes molecular data in the context of the Multiscale Maps to detect significantly perturbed or activated hierarchical components, rather than single genes only, taking into account the type of molecular data, gene size and position, as well as the hierarchical pathway information from the Multiscale Map.
Increasing power
And importantly, these associations can often be made when it would not be possible to make them at the single gene level (due to lack of power). Standard approaches – identifying individual genes mutated at high frequencies – detect many potential drivers but lack power to identify genes mutated less frequently that still may play key roles in cancer (see the comprehensive analysis from Gad Getz lab here).
Importantly, it is often of interest to find the associations between putative drivers and clinical phenotypes such as patient survival, response to treatment or cancer stage. By analyzing molecular and clinical data across over 33 cancers profiled by The Cancer Genome Atlas (TCGA) we found that we are able to detect up to three times more statistically significant associations (while correcting for pathway/gene overlap) with Multiscale Map features rather than single features. Molecular features analyzed include somatic mutations, CNV and DNA hypermethylation events:
Moreover, the number of phenotypes across the 33 cancers for which at least one biomarker candidate was identified increased ~2 fold by including multiscale markers:
For many problems, Multiscale Maps can provide a tool to help address biological complexity and small sample size.
We think it’s time to move toward more comprehensive systems-level views of health and disease. Multiscale Maps provide a tool that makes it easy to explore molecular data and find key insights at any level of molecular hierarchy: from individual genes and proteins, to complexes, pathways and higher-order processes.
Let us know what you think! Drop us a line at info@data4cure.com or request a free demo of the Multiscale Maps and our Biomedical Intelligence Cloud at demo@data4cure.com.
Until next time,
have a great week!
Janusz Dutkowski
Further reading
- Dutkowski et al., Nature Biotechnology 31, 38–45 (2013) doi:10.1038/nbt.2463
- Dutkowski et al., Nucleic Acids Research 42, D1–D6 (2014) doi:10.1093/nar/gkt1192
- Dolinski and Bostein, Nature Biotechnology 31, 34–35 (2013) doi:10.1038/nbt.2476
- Ideker, Dutkowski, Hood, Cell 144(6), 860-3 (2011) doi:10.1016/j.cell.2011.03.007.
- Yu, et al., Cell Systems 2(2): 77–88. (2016) doi:10.1016/j.cels.2016.02.003
- Carter, Hofree, Ideker, Current Opinion in Genetics & Development 23 (6): 611–621. (2013) doi:10.1016/j.gde.2013.10.003
- Carvunis, Ideker, Cell (2014) doi:10.1016/j.cell.2014.03.009